In 2018, I shared the success of a client’s event (Black Girl Convention) with a fellow marketer at a networking event. During the conversation I was met with the question: ,”Must be hard to create content with such a limited audience?” – the limited audience being Black women.
Since founding Arima & Co,statementslike the above,whileshocking,arenotuncommon.Ihavelostcount of the number of timesBlackclientsorIwereadvised:“DonotlimityourbusinessbyonlytargetingBlackpeople”.However,hearingthisfrom a whitemarketeerwhomIknewhadBlackclients and createdcampaignstargetingBlackpeoplemademeuncomfortable.Iconsidermarketers to have the power to change the perception of peoplewithinsociety and hearingsomeonebeingsodismissive of a keysegment of theircustomerbaseangeredme.It is because of suchstatements and my experiences of feelingunrepresentedas a childgrowingupin the UK,thatIbecamecommitted to teachingothermarketersways of beinginclusivewithinmarketing.
My mission is to make inclusive marketing the industry standard, with my research, training and development of tools and resources. The marketing world today is mostly digital which means content creates and produces data. To make my mission a reality we have to start with the data. As the saying goes “If you can’t measure it, you can’t improve it.”
As a marketer, a key common thread within my work is data. Data is involved in each aspect of marketing, from idea to creation to reviewing and consumption. Within my work, I continuously review and assess data to evaluate and support my clients’ content performance, conversions and business growth., conversions and content performance.
The common misconception that data and analytics are objectives, is a thing of the past. We don’t have to look too far in history to find examples where neglect of inclusionary methods has led to data bias in an algorithm. Some of the most innovative companies in the technology industry fail to see data bias when implementing new products.
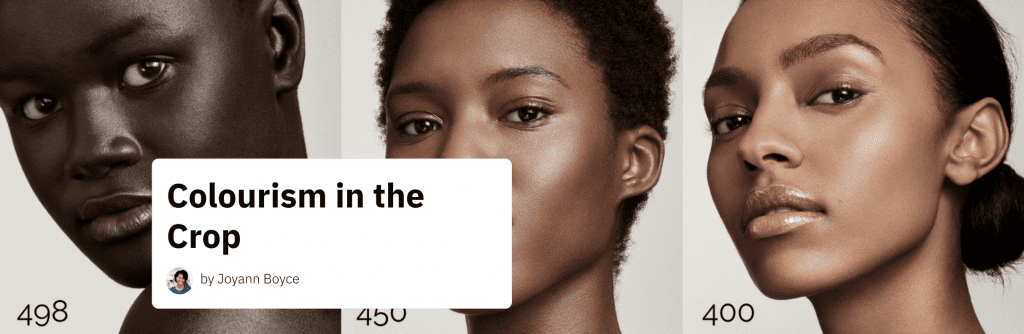
It’s not enough to blame the machines anymore, as these are often extensions of the developers, the product owners, the humans who program them and as such will take on our subjective judgements, whether intentional or not. Let’s not forget that these algorithms are often imagined by a human, developed by a collection of humans and then implemented by humans. We cannot escape this reality, and it led me on this journey to find a solution for data bias.
During the SWCTN Data Fellowship, my key research question was: What if we created an algorithmsolely to benefit Black and Brown people, what would we learn? Specifically, I was interested in exploring how we can learn more about creating unbiased machines by flipping the bias. In other words, we can programme AI in favour of marginalised groups, allowing us to then draw comparisons.
I explored the machine learning process and reviewed at which point the data could be manipulated, the different ways in which data is collected, labelled, cleaned and used to train the machine. A lot of the time bias seems not to be considered until the product is deployed. This exploration also led to my belief that there is no such thing as unbiased data.
The data becomes biased as soon as it’s collected.
I began to think that we could inject positive bias in the earlier stages of idea development. With most products, marketing and business people tend to start with a persona, a fictional character to whom the product or service is meant to be relevant. This is one of the earliest stages in which bias can be manipulated as personas are created with a mixture of data from wider society, the marketers assumptions. These personas are mostly limited to the boundaries of the creator’s imagination and can be burdened by stereotypical traits, be it intentional or not.
Keeping the following factors in mind:
- a persona can be developed by one person or a team of 20+
- it may be fully fictional with no data information or be fully backed by direct user data analytics
- the creators of the persona may have never considered the bias they bring to the table
- sometimes the persona will be focused around the demographic and data of marginalised people
I want to be able to provoke thought in a flexible aspect. I want marketers to think of personas in a deeper and more complex way, to think of and factor in the bias that already exists in society. Create personas with conscious bias. Going back to my early example of my client, Black Girl Convention, I created such a persona at that time, one I would now describe as, An Intersectional Persona.
First coined by Professor Kimberlé Crenshaw back in 1989, intersectionality is a framework to understand how different aspects of a person’s identity can combine to create discrimination or privilege. On this basis, I created an Intersectional Persona Shuffle tool (with development support from Tessa Alexander).
The Persona Shuffle asks the user two sets of questions. They are first shown three consecutive images of faces and asked under each ‘Could this person be your customer?’. The images of faces used were created by an AI and are not of real people. They are then given options of ‘Yes’ or ‘No’ and response time is measured. For every user 2 of the 3 faces is a person of colour. The next 3 questions ask ‘Have you considered that your ideal customer could be?’, showing the user intersectional persona descriptions such as ‘Someone of the Buddhist faith, who is Female, may have a loss of Sight, is Heterosexual and is between 45-64 years old with this
#804e31
skin tone’. Their time to respond is also measured along with which characteristic receives a higher negative or positive response.
This tool does not measure whether the user picks ‘yes’ or ‘no’ but the length of time they take to answer. The theory is that the longer they take the more likely they have not considered some aspect of the question. Over the fellowship, I have used the tool in workshops and the most common response has been ‘We never thought about X aspect’ to make our content inclusive.
Lessons learnt & future work
The data fellowship supported me to explore ways in which negative or positive bias can be manipulated in the early stages of idea development. My next step was to explore how it could be identified and flagged in the creation stage. To continue my research I applied for the Data Prototype grant by SWCTN and enrolled on a Data Science Masters at the University of the West of England. I was privileged to be awarded grants for both and be able to continue my research and build a prototype of my idea. That prototype, Includ, aims to be the next step in bias awareness in content creation, allowing a user to upload marketing content and receive a report of potential areas of bias. If a human can create AI to replicate the bias we hold in society why can’t we create AI to identify and redress the bias?
Resources Discovered
People of influence
A Twitter List of some of the influential people I discovered, some of which are:
- Kate Crawford
- Ruha Benjamin
- Joy Buolamwini
- Cathy O’Neil
- Yeshimabeit Milner (Data 4Blakc Lives)
- Daniel Whitenack
- Dr Francesca Sobande
Things to watch
Ted talks
- How I’m fighting bias in algorithms by Joy Buolamwini
- The Era of blind faith in big data must end by Cathy O’Neil
- Your social media “likes” expose more than you think by Jennifer Golbeck
- The Birth of a word by Deb Roy
- How I hacked online dating by Amy Webb
- 3 ways to spot bad data by Mona Chaalabi
Films
- Coded Bias
- Netflix Connected: The Hidden Science of Everything – Ep.4 Digits
- Netflix : The Social Dilemma
- Netflix: The Great Hack
Talks & Conferences
- Data & Human
- Open UP Summit
- Turning the tables on Facebook: How we audit Facebook using their own marketing tools
To Listen
To read
- Data Feminism by Catherine D`ignazio, Lauren F. Klein
- Invisible Women: Exposing Data Bias in a World Designed for Men by Caroline Criado Perez
- Algorithms of Oppression: How Search Engines Reinforce Racism by Safiya Umoja Noble
- The Age of Surveillance Capitalism by Shoshana Zuboff
- Dark Matters by Simone Browne
- Race After Technology: Abolitionist Tools for the New Jim Code by Ruha Benjamin
- The Intersectional Internet: Race, Sex, Class, and Culture Online (Digital Formations) by Safiya Umoja Noble
- Critical Race Theory: An Introduction by Richard Delgado, Jean Stefancic and Angela Harris
- Superior: The Return of Race Science by Angela Saini